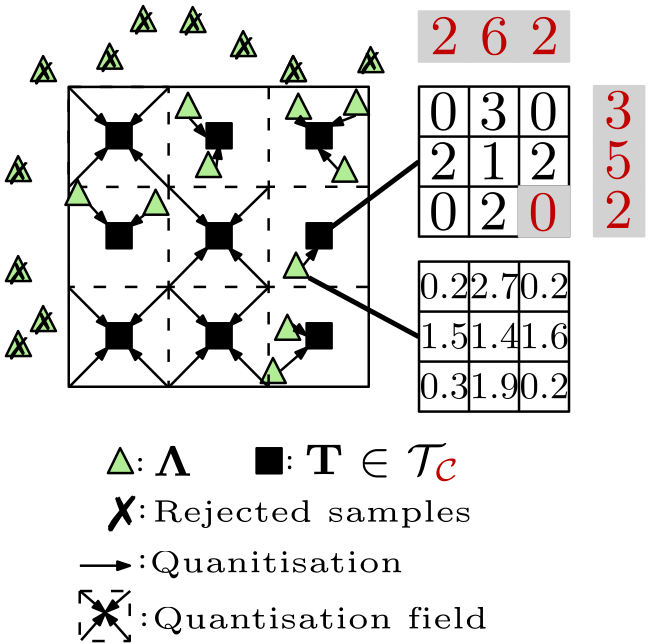
Generating Origin-Destination Matrices in Neural Spatial Interaction Models
9 September 2024
Agent-based models (ABMs) are proliferating as decision-making tools across policy areas in transportation, economics, and epidemiology. In these models, a central object of interest is the discrete origin-destination matrix which captures spatial interactions and agent trip counts between locations. Existing approaches resort to continuous approximations of this matrix and subsequent ad-hoc discretisations in order to perform ABM simulation and calibration. This impedes conditioning on partially observed summary statistics, fails to explore the multimodal matrix distribution over a discrete combinatorial support, and incurs discretisation errors.
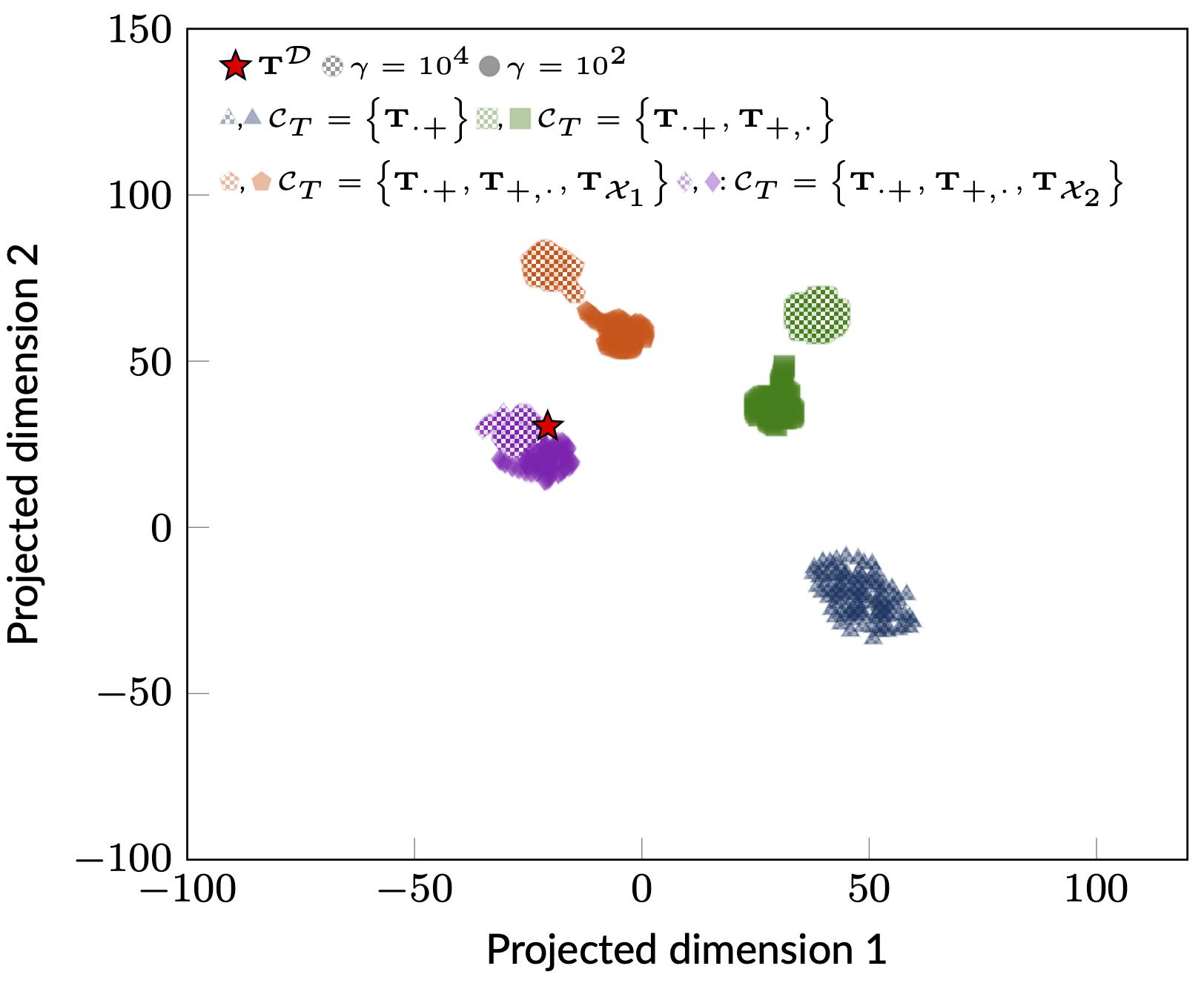
Table inference for combinatorial origin-destination choices in agent-based population synthesis
7 July 2023
A key challenge in agent-based mobility simulations is the synthesis of individual agent socioeconomic profiles. Such profiles include locations of agent activities, which dictate the quality of the simulated travel patterns. These locations are typically represented in origin-destination matrices that are sampled using coarse travel surveys. This is because fine-grained trip profiles are scarce and fragmented due to privacy and cost reasons. The discrepancy between data and sampling resolutions renders agent traits non-identifiable due to the combinatorial space of data-consistent individual attributes.
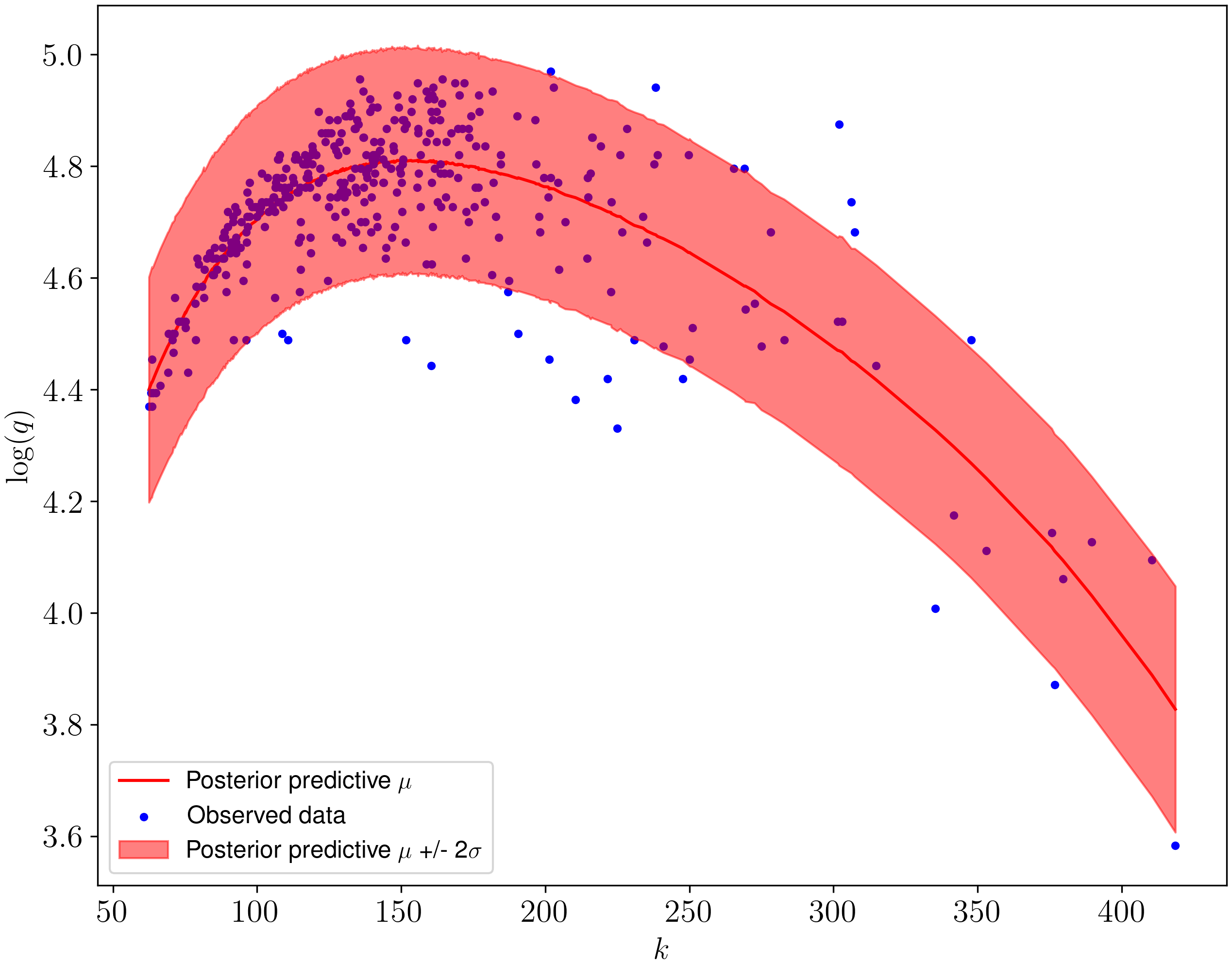
Model assessment of constitutive laws in traffic conservation laws
10 October 2021
The proliferation of traffic sensing devices on motorways has spurred interest in data assimilation in macroscopic traffic flow partial differential equations, such as the Lighthill Whitham Richards (LWR) model. The empirical constitutive laws (fundamental diagrams) embedded within these PDEs are uncertain and misspecified. We leverage a Bayesian model assessment framework to robustly identify the most plausible FDs accounting for the trade-off between model fit and complexity. This is achieved by computing unbiased estimates of the marginal likelihood for each FD based on ideas from thermodynamic integration while sampling from the resulting power posteriors using a Metropolis Hastings Markov Chain Monte Carlo algorithm.
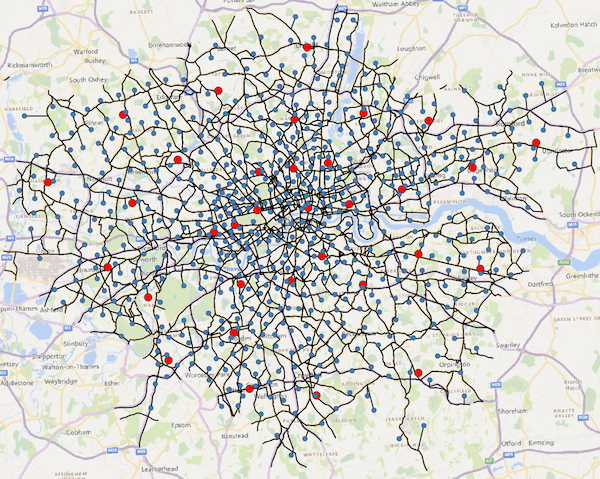
Stochastic modelling of urban travel demand
8 August 2020
Modelling of evolution of urban travel demand is fundamental for urban planners and policy makers to assess the spatial demand for transportation capacity and decide on appropriate interventions. We follow the approach of (Ellam et al., 2018) and introduce a novel application of spatial interaction models and a mathematical evolution of their dynamics to urban travel demand. We exploit economic structure characteristics (e.g. employment) to inform travel demand between a set of origin and destination locations.
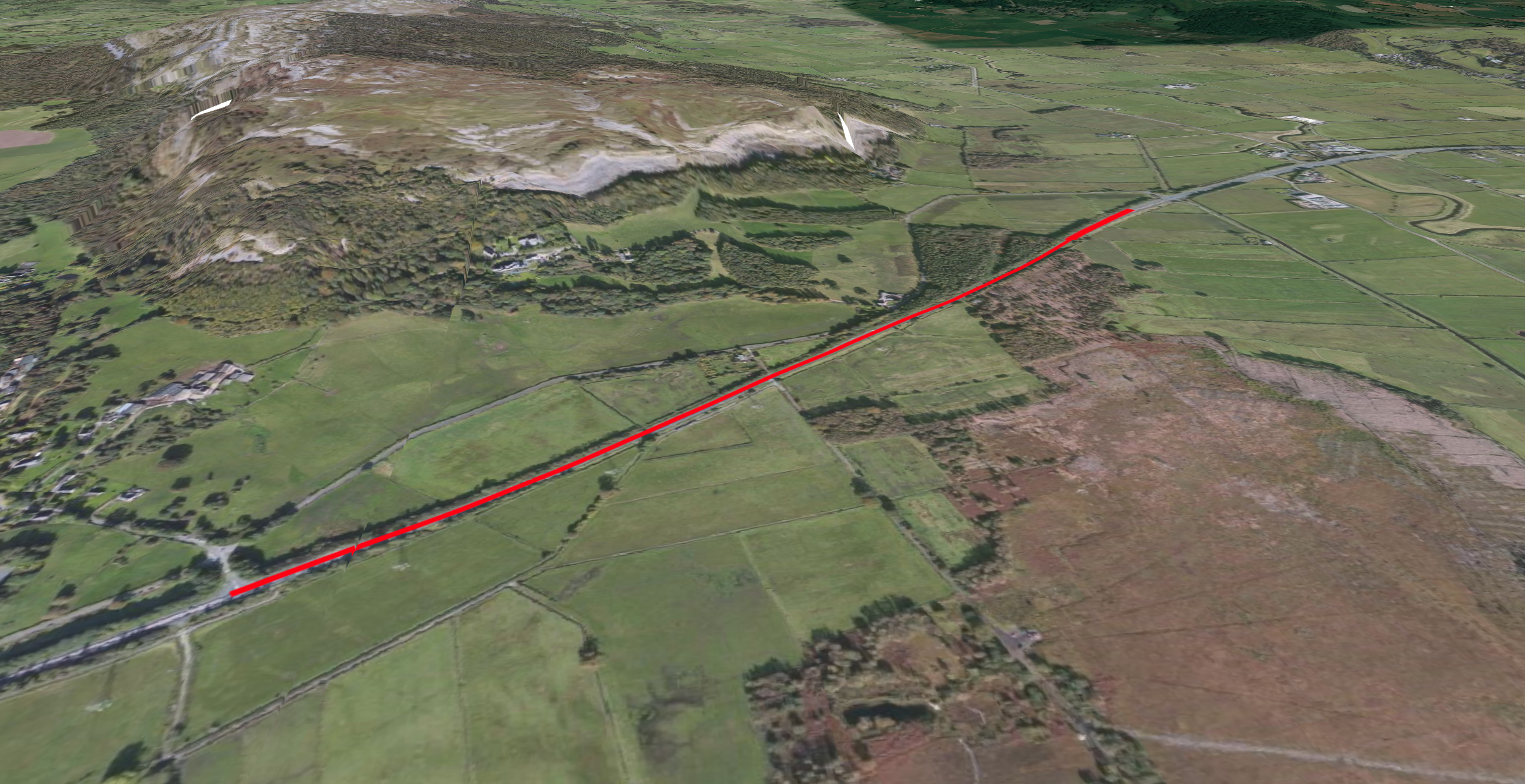
Bayesian hydrological modelling of road rainfall run-off
1 January 2020
National Highway’s objective to improve the health and safety of its customers is tightly linked to better monitoring of on-road water discharge. The increasing climate volatility can impede accurate hydrological modelling and therefore a probabilistic approach is adopted to modelling on-road rainfall-runoff. This report illustrates the potential of using a hybrid of statistical and hydrological models to better understand on-road water conditions. The study area is a major A-road in north-west of England.
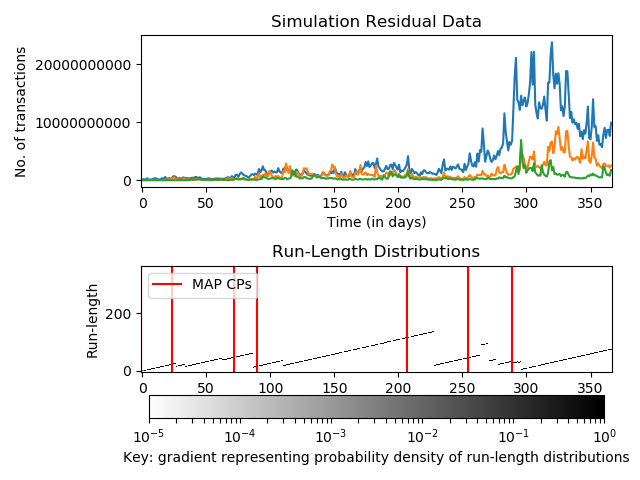
Bayesian online changepoint detection of point processes
6 June 2018
This thesis details an approach known as change-point detection (CPD) that aims to detect changes in the mean, variance and covariance of a time series. The scope of CPD is limited to an on-line (real-time) Bayesian spatio-temporal setting. In this setting, the goal of CPD is to provide step-ahead predictions and partition the time series into disjoint segments every time a new datum is received using Bayesian inference. This is achieved by modelling each datum as a sample from a data-generating process which we are imitating using a probability distribution as a model.